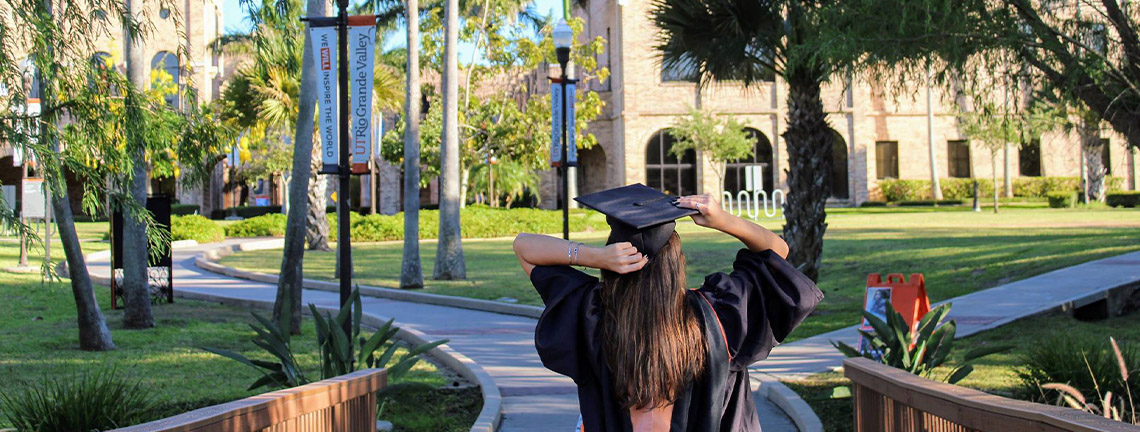
Theses and Dissertations
Date of Award
8-2023
Document Type
Thesis
Degree Name
Master of Science (MS)
Department
Computer Science
First Advisor
Dong-Chul Kim
Second Advisor
Erik Enriquez
Third Advisor
Emmett Tomai
Abstract
Humans possess an extraordinary ability to execute complex movements, captivating the attention of researchers who strive to develop methods for simulating these actions within a physics-based environment. Motion Capture data stands out as a crucial tool among the proven approaches to tackle this challenge. In this research, we explore the effects of decreased muscle force on the body's capacity to perform various tasks, ranging from simple walking to executing complex jumping jacks. Through a systematic reduction of the allowed force applied to individual muscles or muscle groups, we aim to identify the threshold at which the body's muscles tolerate the deficiency before the motion becomes unattainable. Additionally, we seek to analyze how the model adapts its muscle activation levels, in scenarios where the motion is still achievable despite the applied deficiencies.
Recommended Citation
Castillo, Daniel, "Simulating Motion Success With Muscle Deficiency in a Musculoskeletal Model Using Reinforcement Learning" (2023). Theses and Dissertations. 1325.
https://scholarworks.utrgv.edu/etd/1325
Comments
Copyright 2023 Daniel Castillo. All Rights Reserved.
https://go.openathens.net/redirector/utrgv.edu?url=https://www.proquest.com/dissertations-theses/simulating-motion-success-with-muscle-deficiency/docview/2862069195/se-2?accountid=7119