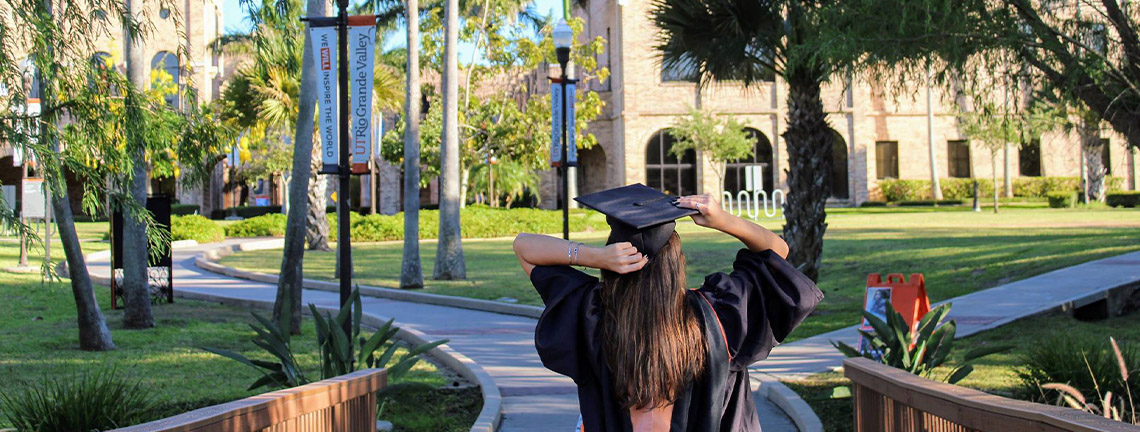
Theses and Dissertations
Date of Award
8-2023
Document Type
Thesis
Degree Name
Master of Science in Engineering (MSE)
Department
Mechanical Engineering
First Advisor
Constantine Tarawneh
Second Advisor
Mohamadhossein Noruzoliaee
Third Advisor
Fatemeh Nazari
Abstract
One of the primary causes of rail rolling stock derailments is attributed to bearing and wheel axle failures. The health of train bearings is primarily monitored at target locations through wayside detection systems. This practice is susceptible to bearing failure and potential derailments at points in between these wayside systems. To remedy this, the University Transportation Center for Railway Safety (UTCRS) has developed a wireless onboard monitoring system that can continuously monitor the vibration response, which directly correlates to the health of bearings. This data is used to train regression-based machine learning algorithms and long-term prediction neural networks to predict bearing health. The models are intended to work in tandem with the onboard monitoring sensors as a means of two-way practical validation. The models tested were the Gradient Boosting Machine architecture for scheduled predictions and the Informer neural network architecture for long-term predictions of ongoing routes. The dataset for these models comes from the expansive laboratory record data available at the UTCRS. Ultimately, these machine learning algorithms will enhance freight railcars safety and save rail companies money by allowing for predictive maintenance practices.
Recommended Citation
Martinez, Sergio M., "Vibration-Based Machine Learning Models for Condition Monitoring of Railroad Rolling Stock" (2023). Theses and Dissertations. 1368.
https://scholarworks.utrgv.edu/etd/1368
Comments
Copyright 2023 Sergio M. Martinez. All Rights Reserved.
https://go.openathens.net/redirector/utrgv.edu?url=https://www.proquest.com/dissertations-theses/vibration-based-machine-learning-models-condition/docview/2863126376/se-2?accountid=7119