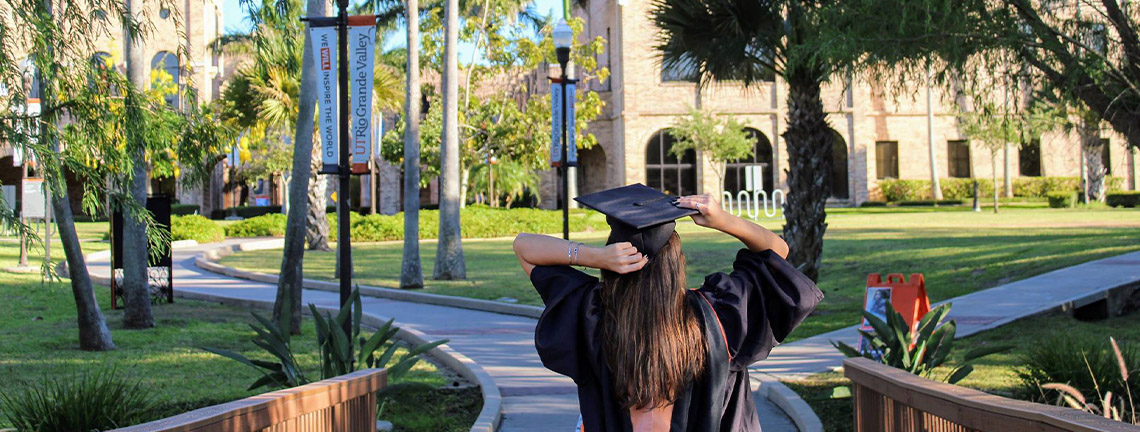
Theses and Dissertations
Date of Award
7-2023
Document Type
Thesis
Degree Name
Master of Science (MS)
Department
Physics
First Advisor
Mario C. Diaz
Second Advisor
Liliana Rivera Sandoval
Third Advisor
Nicolas Pereyra
Abstract
We present a technique for optical transient detection using artificial neural networks, particularly a Convolutional Neural Network (CNN), a deep learning algorithm. This method analyzes images of the same area of the sky captured by several telescopes, with one image serving as a reference for a probable transient’s epoch and the other as an image from a previous epoch. We train the CNN on simulated sources and test it on actual image data samples using data from the Dr. Cristina V. Torres Memorial Astronomical Observatory and Sloan Digital Sky Survey. This autonomous detection method replaces the standard procedure, which involves source extraction from a different image and subsequent human inspection. Automating the pro- cess enables faster, more efficient follow-ups on intriguing targets. For greater accessibility, we increase the CNN algorithm’s datasets and incorporate them into a user-friendly web application. Future expansion and testing will be conducted with telescopes from the Transient Optical Robotic Observatory of the South Collaboration, further refining the detection process for optical astronomical transients.
Recommended Citation
Mendoza, Wendy, "Optimizing Convolutional Neural Networks for Transient Detection in Optical Astronomy With Augmented Datasets" (2023). Theses and Dissertations. 1372.
https://scholarworks.utrgv.edu/etd/1372
Comments
Copyright 2023 Wendy Mendoza. All Rights Reserved.
https://go.openathens.net/redirector/utrgv.edu?url=https://www.proquest.com/dissertations-theses/optimizing-convolutional-neural-networks/docview/2862098757/se-2?accountid=7119