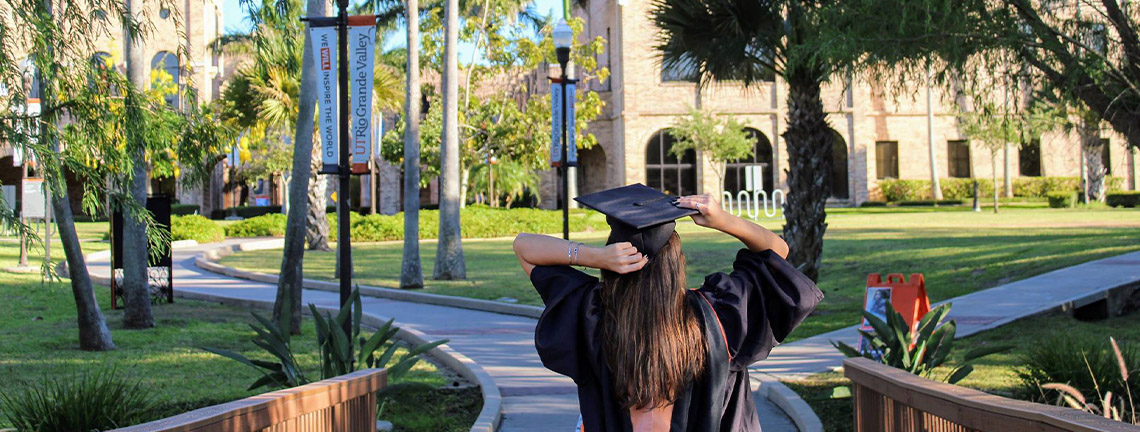
Theses and Dissertations
Date of Award
7-2023
Document Type
Thesis
Degree Name
Master of Science (MS)
Department
Applied Statistics and Data Science
First Advisor
Hansapani Rodrigo
Second Advisor
Tamer Oraby
Third Advisor
Michael Machiolatti
Abstract
This project is an application of the Symbolic Aggregate Approximation (SAX) to 1000 fragments of ECG signals for 45 patients (42% females aged between 23 and 89 years and 58% males aged 32 to 89 years) using data obtained from the MIH-BIH Arrhythmia database to recognize cardiac health disorders. Data include a normal sinus rhythm, pacemaker rhythm and ECG readings for 15 heart disorders, making 17 in total. The aim is to use SAX to classify heart disorders using ECG signal, that analyzes QRS-complexes by first splitting the time series into smaller equally sized segments using the Piecewise Aggregate Approximation (PAA) approach. SAX is then used to reduce the normalized PAA series to a string of arbitrary length alphabets. Using a sliding window algorithm, we create a list of word bags that are the result of SAX on our data set – this gives the words as well as frequency counts. We then specify the PAA size, window size and SAX alphabet size, and compute weights for these word bags that give an indication of the proportion of these words sequence in our input time series. This will be done to the sample data set for each heart disorder and at the end, we are able to determine which exact patterns contributes the most (motifs) and the least (discords) to our class of words, facilitating the identification of possible heart disorders.
Recommended Citation
Owusu, M. K. (2023). Identification of Heart Disorders With Symbolic Aggregate Approximation [Master's thesis, The University of Texas Rio Grande Valley]. ScholarWorks @ UTRGV. https://scholarworks.utrgv.edu/etd/1383
Comments
Copyright 2023 Moses K. Owusu. All Rights Reserved.
https://go.openathens.net/redirector/utrgv.edu?url=https://www.proquest.com/dissertations-theses/identification-heart-disorders-with-symbolic/docview/2862107393/se-2?accountid=7119