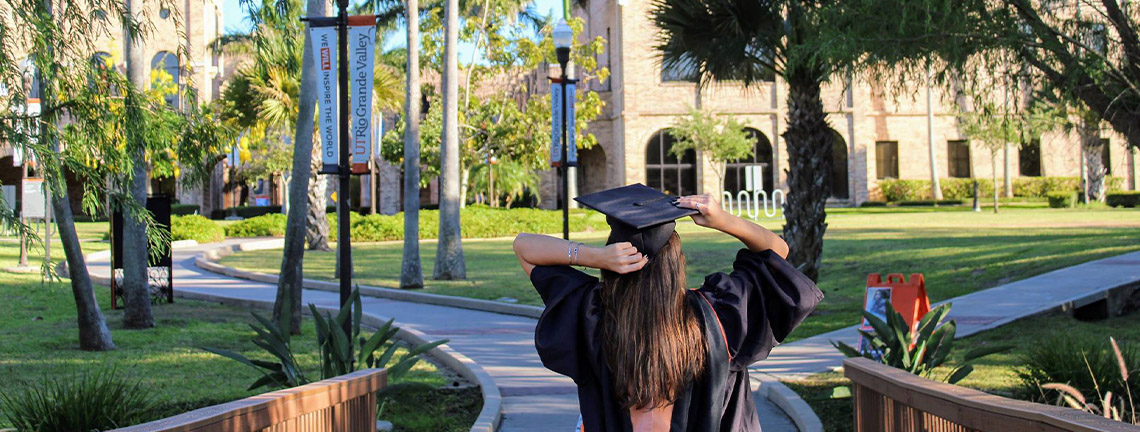
Theses and Dissertations
Date of Award
5-2024
Document Type
Thesis
Degree Name
Master of Science (MS)
Department
Civil Engineering
First Advisor
Mohsen Amjadian
Second Advisor
Philip Park
Third Advisor
Constantine M. Tarawneh
Abstract
The U.S. rail network is among the world's largest, safest, and most efficient. However, many its railway bridges, which play an essential role in its connectivity, are over 100 years old, showing signs of structural deterioration exceeding their practical service life. The increased weight and speed of modern trains also pose a risk to these bridges. Traditional load identification and monitoring technologies like Weigh-in-Motion (WIM) systems are rarely used on railway bridges due to their cost and practical limits. This thesis explores a cost-effective approach to utilize vibration sensors on railway bridges to identify and predict train load features (speed and axle load), using machine learning (ML). The study involves finite element modeling of railway bridges, followed by applying ML algorithms like LSTM and RNN to estimate axle loads and speeds from vibration data. The results shows the acceptable performance of developed time series ML models in predicting these parameters.
Recommended Citation
Rahman, Md Masnun, "Machine Learning Predictive Models for Load Identification of Railroad Bridges Subjected to Train-Induced Vibration" (2024). Theses and Dissertations. 1488.
https://scholarworks.utrgv.edu/etd/1488
Comments
Copyright 2024 Md Masnun Rahman.
https://go.openathens.net/redirector/utrgv.edu?url=https://www.proquest.com/pqdtglobal1/dissertations-theses/machine-learning-predictive-models-load/docview/3085314690/sem-2?accountid=7119