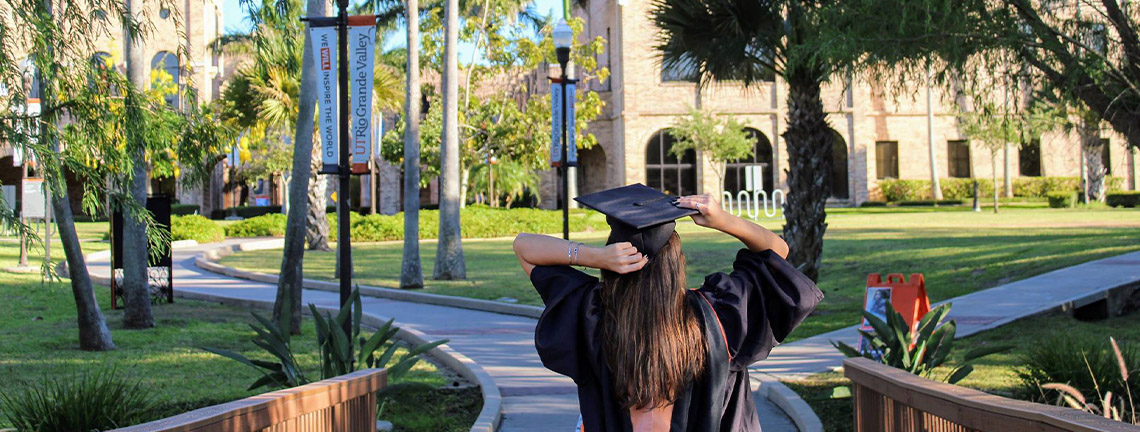
Theses and Dissertations
Date of Award
7-31-2024
Document Type
Thesis
Degree Name
Master of Science (MS)
First Advisor
Hansheng Lei
Second Advisor
Liyu Zhang
Third Advisor
Bin Fu
Abstract
By perturbation or physical attacks any machine can be fooled into predicting something else other than the intended output. There are training data based on which the model is trained to predict unknown things. The objective was to create noises and shades of different levels on the images and do experiments for measuring accuracy and making the model classify the traffic signs. When it comes to adding shades to the pictures, pixels were modified for three different layers of the pictures. The experiment also shows that with the shadows getting deeper, the accuracies drop significantly. Here, some changes in pixels are being made, which is referred to as perturbation. The perturbation may not be perceived by human beings, but the computer systems can perceive the differences.
Our experiments display that using perturbations, the pictures of the traffic signs could be changed, and the machine displayed that the accuracies getting changed in both the scenarios, first, the noise being added to the pictures and then the shadows being created in the pictures.
Recommended Citation
Mukhopadhyay, Gourab, "Increasing the Robustness of Machine Learning by Adversarial Attacks" (2024). Theses and Dissertations. 1496.
https://scholarworks.utrgv.edu/etd/1496
Comments
Copyright 2024 Gourab Mukhopadhyay.
https://go.openathens.net/redirector/utrgv.edu?url=https://www.proquest.com/pqdtglobal1/dissertations-theses/increasing-robustness-machine-learning/docview/3085720622/sem-2?accountid=7119