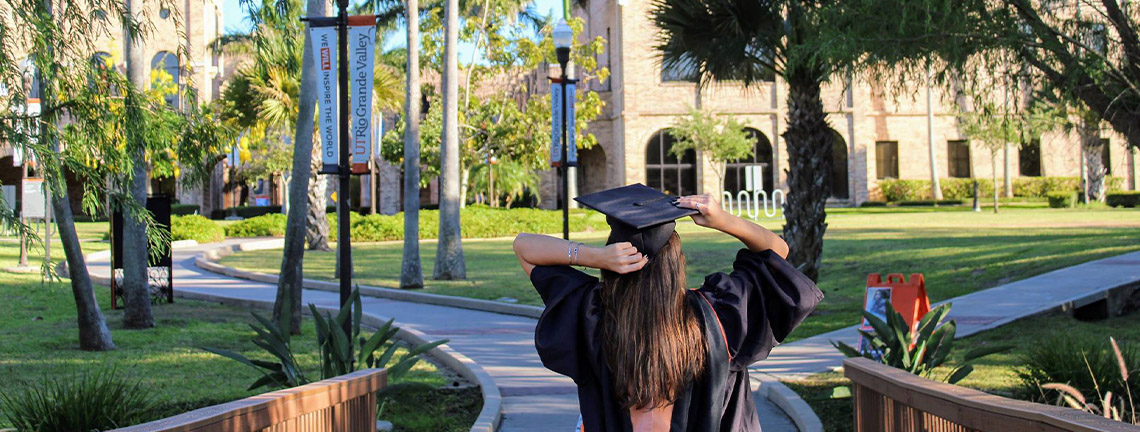
Theses and Dissertations
Date of Award
8-1-2024
Document Type
Dissertation
Degree Name
Doctor of Philosophy (PhD)
Department
Mathematics
First Advisor
Haoyuan Chen
Second Advisor
Zhijun Qiao
Third Advisor
Hansapani Rodrigo
Abstract
Metal-organic frameworks (MOFs) are nanoporous materials with a structure and chemistry that can be tailored with precision to support a variety of applications, such as gas storage, drug delivery, catalysis, and sensing. Central to the realization of these applications is the need to model adsorption in MOFs. However, if the goal is to screen a large number of the hundreds of thousands of MOFs discovered, conventional high-throughput molecular simulations are prohibitively demanding in terms of computational resources. Therefore, efficient approaches are required to efficiently identify suitable MOFs for specific applications. In our work, machine learning (ML) models to predict adsorption of nitrogen and carbon dioxide in MOFs are tested. The comparison of three main ML paradigms (linear regression, neural networks and tree-based algorithms) together with an appropriate feature engineering setup, shows that modeling can be performed efficiently and with notable accuracy. The features used for modeling possess physical interpretation and can be meaningfully measured against ML predictions using SHAP values as a measure of feature importance, providing insights that align with first-principles adsorption mechanisms.
Recommended Citation
Benitez, J. G. (2024). Benchmarking Machine Learning Algorithms for the Prediction of Gas Adsorption in Metal-Organic Framework Materials [Doctoral dissertation, The University of Texas Rio Grande Valley]. ScholarWorks @ UTRGV. https://scholarworks.utrgv.edu/etd/1585
Comments
Copyright 2024 Juan G Benitez. https://proquest.com/docview/3098420242