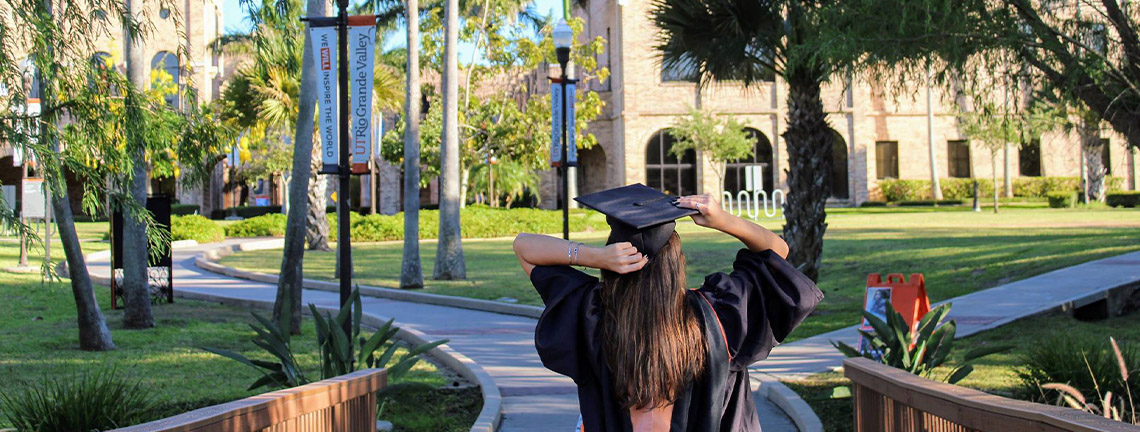
Theses and Dissertations
Date of Award
8-1-2024
Document Type
Thesis
Degree Name
Master of Science (MS)
First Advisor
Hansheng Lei
Second Advisor
Liyu Zhang
Third Advisor
Jorge Castillo
Abstract
The maritime industry relies on the Automated Identification System (AIS) for real-time vessel tracking and navigation. This thesis focuses on detecting vessel delays and mining delay patterns from AIS data, which are crucial for improving shipping schedules, port operations, and global trade logistics. The research begins with rigorous data preprocessing, including noise reduction, data interpolation, and anomaly detection, to ensure high-quality AIS data as well as focuses on detecting vessel delays and mining delay patterns from AIS data to enhance maritime operations. Advanced machine learning models analyze temporal and spatial features to detect delays, considering vessel type, speed, and route. Pattern mining techniques, such a time-series analysis, identify recurring delay patterns and their causes, including weather conditions, port congestion, and navigational challenges. The findings offer actionable insights for maritime stakeholders, improving decision-making and operational efficiency. The developed methodologies can be integrated into existing monitoring systems for real-time delay predictions, contributing to the optimization of global maritime logistics.
Recommended Citation
Ara, Naznin, "Vessel Delay Detection and Pattern Mining From AIS Data" (2024). Theses and Dissertations. 1617.
https://scholarworks.utrgv.edu/etd/1617
Comments
Copyright 2024 Naznin Ara. https://proquest.com/docview/3115240381