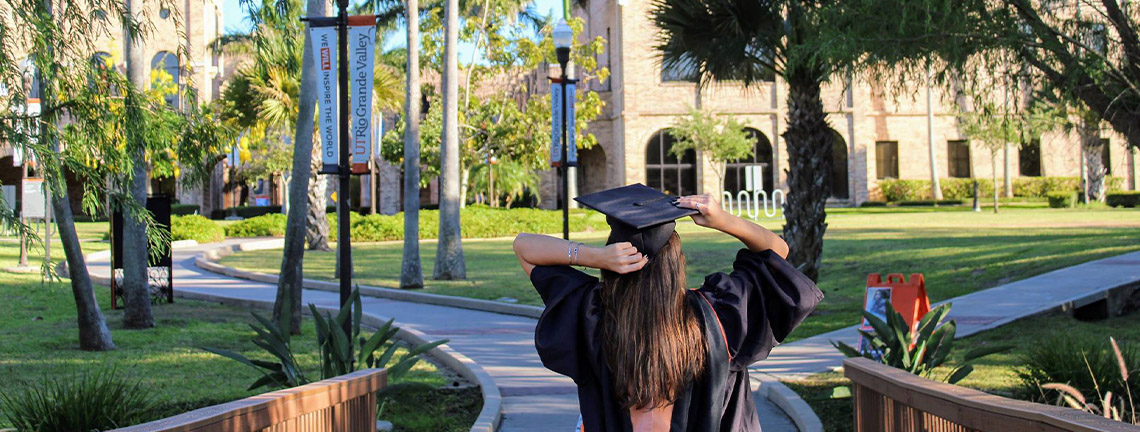
Theses and Dissertations
Date of Award
12-2019
Document Type
Thesis
Degree Name
Master of Science (MS)
Department
Computer Science
First Advisor
Dr. Hongkai Yu
Second Advisor
Dr. Jingru Zhang
Third Advisor
Dr. Bin Fu
Abstract
The field of deep learning has experienced a resurgence in the recent years, particularly resulting with the advent of AlexNet. Supervised learning is currently the most common and practical machine learning method. The struggle with employing supervised learning to approach problems is that it requires training data. Sufficient training data is correlated with performance for deep learning models. The issue is that preparing the training data can be a tedious and labor intensive task, especially on a large scale. The purpose of this paper is to determine how efficient a machine can learn when trained on automatically annotated data. The goal is to determine whether or not automatically generated training data is viable for teaching machines. This proposed framework is designed to generate data and train a classifier for pedestrian detection. The framework will be evaluated on a tailored pedestrian dataset and PASCAL VOC 2007.
Recommended Citation
Chen, Andrew Y., "Learning to Detect Pedestrians by Watching Videos" (2019). Theses and Dissertations. 436.
https://scholarworks.utrgv.edu/etd/436
Comments
Copyright 2019 Andrew Y. Chen. All Rights Reserved.
https://go.openathens.net/redirector/utrgv.edu?url=https://www.proquest.com/dissertations-theses/learning-detect-pedestrians-watching-videos/docview/2382602288/se-2?accountid=7119