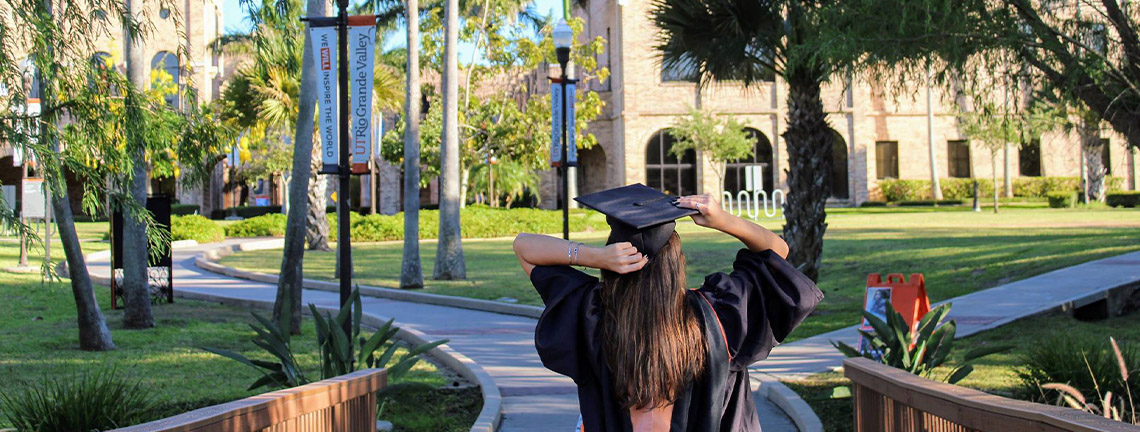
Theses and Dissertations
Date of Award
8-2020
Document Type
Thesis
Degree Name
Master of Science (MS)
Department
Electrical Engineering
First Advisor
Dr. Yong Zhou
Second Advisor
Dr. Samir Iqbal
Third Advisor
Dr. Nazmul Islam
Abstract
The Master’s thesis is comprised of four projects in the realm of machine learning and signal processing. The abstract of the thesis is divided into four parts and presented as follows,
Abstract 1: A Kullback-Leibler Divergence-Based Predictor for Inter-Subject Associative BCI.
Inherent inter-subject variability in sensorimotor brain dynamics hinders the transferability of brain-computer interface (BCI) model parameters across subjects. An individual training session is essential for effective BCI control to compensate for variability. We report a Kullback-Leibler Divergence (KLD)-based predictor for inter-subject associative BCI. An online dataset comprising left/right hand, both feet, and tongue motor imagery tasks was used to show correlation between the proposed inter-subject predictor and BCI performance. Linear regression between the KLD predictor and BCI performance showed a strong inverse correlation (r = -0.62). The KLD predictor can act as an indicator for generalized inter-subject associative BCI designs.
Abstract 2: Multiclass Sensorimotor BCI Based on Simultaneous EEG and fNIRS.
Hybrid BCI (hBCI) utilizes multiple data modalities to acquire brain signals during motor execution (ME) tasks. Studies have shown significant enhancements in the classification of binary class ME-hBCIs; however, four-class ME-hBCI classification is yet to be done using multiclass algorithms. We present a quad-class classification of ME-hBCI tasks from simultaneous EEG-fNIRS recordings. Appropriate features were extracted from EEG-fNIRS signals and combined for hybrid features and classified with support vector machine. Results showed a significant increase in hybrid accuracy over single modalities and show hybrid method’s performance enhancement capability.
Abstract 3: Deep Learning for Improved Inter-Subject EEG-fNIRS Hybrid BCI Performance.
Multimodality based hybrid BCI has become famous for performance improvement; however, the inherent inter-subject and inter-session variation between participants brain dynamics poses obstacles in achieving high performance. This work presents an inter-subject hBCI to classify right/left-hand MI tasks from simultaneous EEG-fNIRS recordings of 29 healthy subjects. State-of-art features were extracted from EEG-fNIRS signals and combined for hybrid features, and finally, classified using deep Long short-term memory classifier. Results showed an increase in the inter-subject performance for the hybrid system while making the system more robust to brain dynamics change and hints to the feasibility of EEG-fNIRS based inter-subject hBCI.
Abstract 4: Microwave Based Glucose Concentration Classification by Machine Learning.
Non-invasive blood sugar measurement attracts increased attention in recent years, given the increase in diabetes-related complications and inconvenience in the traditional ways using blood. This work utilized machine learning (ML) algorithms to classify glucose concentration (GC) from the measured broadband microwave scattering signals (S11). An N-type microwave adapter pair was utilized to measure the sweeping frequency scattering-parameter (S-parameter) of the glucose solutions with GC varying from 50-10,000 dg/dL. Dielectric parameters were retrieved from the measured wideband complex S-parameters based on the modified Debye dielectric dispersion model. Results indicate that the best algorithm can achieve a perfect classification accuracy and suggests an alternate way to develop a GC detection method using ML algorithms.
Recommended Citation
Hossain, Md Shakhawat, "Signal Processing Combined with Machine Learning for Biomedical Applications" (2020). Theses and Dissertations. 680.
https://scholarworks.utrgv.edu/etd/680
Comments
Copyright 2020 Md Shakhawat Hossain. All Rights Reserved.
https://go.openathens.net/redirector/utrgv.edu?url=https://www.proquest.com/dissertations-theses/signal-processing-combined-with-machine-learning/docview/2496218341/se-2?accountid=7119