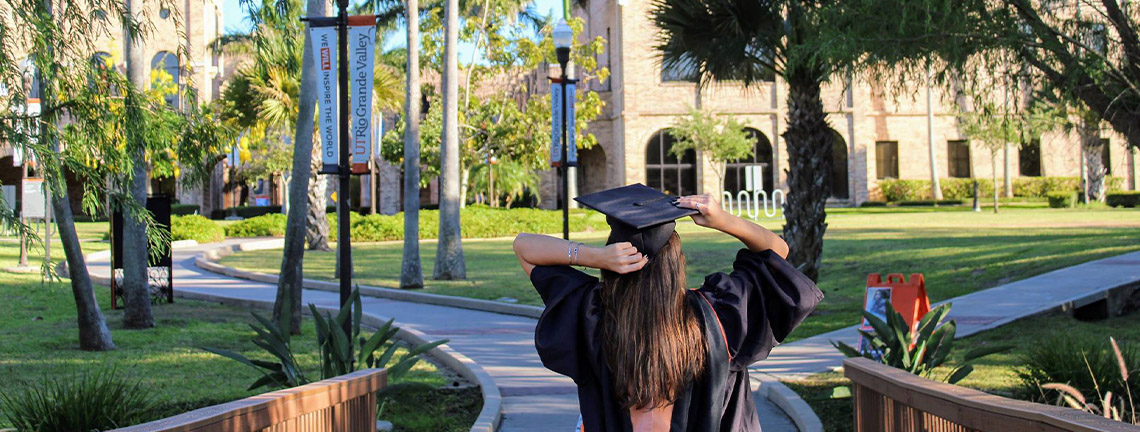
Theses and Dissertations
Date of Award
12-2020
Document Type
Thesis
Degree Name
Master of Science (MS)
Department
Civil Engineering
First Advisor
Dr. Mohamadhossein Noruzoliaee
Second Advisor
Dr. Fatemeh Nazari
Third Advisor
Dr. Mohamed Abdel Raheem
Abstract
In the modern world of Intelligent Transportation System (ITS), time headway is a key traffic flow parameter affecting ITS operations and planning. Defined as “the time difference between any two successive vehicles when they cross a given point”, time headway is used in various traffic and transportation engineering research domains, such as capacity analysis, safety studies, car-following, and lane-changing behavior modeling, and level of service evaluation describing stochastic features of traffic flow. Advanced travel and headway information can also help road users avoid traffic congestion through dynamic route planning, for instance. Hence, it is crucial to accurately model headway distribution patterns for the purpose of analyzing traffic operations and making subsequent infrastructure-related decisions. Previous studies have applied a variety of probabilistic models, machine learning algorithms (for example, support vector machine, relevance vector machine, etc.), and neural networks for short-term headway prediction. Recently, deep learning has become increasingly popular following a surge of traffic big data with high resolution, thriving algorithms, and evolved computational capacity. However, only a few studies have exploited this emerging technology for headway prediction applications. This is largely due to the difficulty in capturing the random, seasonal, nonlinear, and spatiotemporal correlated nature of traffic data and asymmetric human driving behavior which has a significant impact on headway. This study employs a novel architecture of deep neural networks, Long Short-Term Neural Network (LSTM NN), to capture nonlinear traffic dynamics effectively to predict vehicle headway. LSTM NN can overcome the issue of back-propagated error decay (that is, vanishing gradient problem) existing in regular Recurrent Neural Network (RNN) through memory blocks which is its special feature, and thus exhibits superior capability for time series prediction with long temporal dependency.
There is no existing appropriate model for long term prediction of traffic headway, as existing models lack using big dataset and solving the vanishing gradient problem because of not having a memory block. To overcome these critics and fill the gaps in previous works, multiple LSTM layers are stacked to incorporate temporal information. For model training and validation, this study used the USDOT’s Next Generation Simulation (NGSIM) dataset, which contains historical data of some important features to describe the headway distribution such as lane numbers, microscopic traffic flow parameters, vehicle and road shape, vehicle type, and velocity. LSTM NN can capture the historical relationships between these variables and save them using its unique memory block. At the headway prediction stage, the related spatiotemporal features from the dataset (HighwayI-80) were fed into a fully connected layer and again tested with testing data for validation (both highway I-80 & US 101). The predicted accuracy outperforms previous time headway predictions.
Recommended Citation
Masud, Saumik Sakib Bin, "Traffic Time Headway Prediction and Analysis: A Deep Learning Approach" (2020). Theses and Dissertations. 719.
https://scholarworks.utrgv.edu/etd/719
Comments
Copyright 2020 Saumik Sakib Bin Masud. All Rights Reserved.
https://go.openathens.net/redirector/utrgv.edu?url=https://www.proquest.com/dissertations-theses/traffic-time-headway-prediction-analysis-deep/docview/2556342315/se-2?accountid=7119