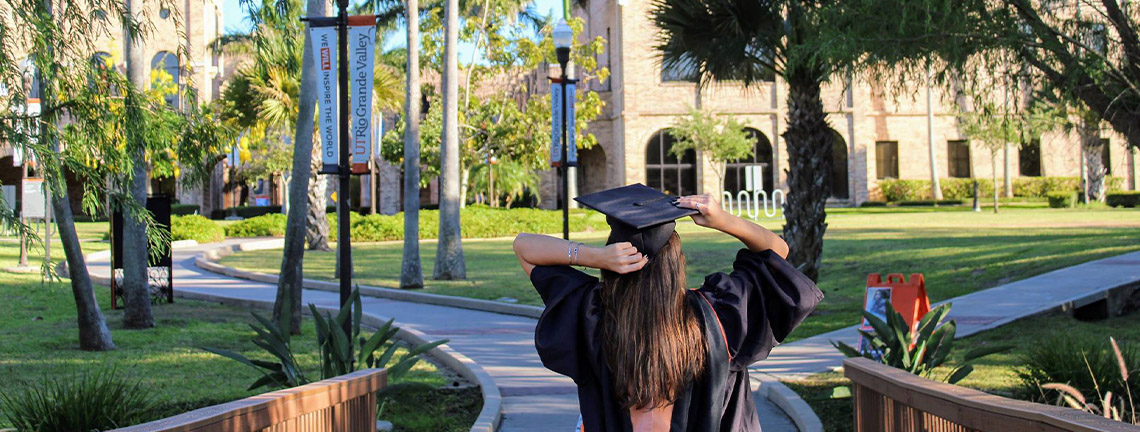
Theses and Dissertations
Date of Award
7-2020
Document Type
Thesis
Degree Name
Master of Science (MS)
Department
Physics
First Advisor
Dr. Mario C. Díaz
Second Advisor
Dr. Juan Madrid
Third Advisor
Dr. Efrain J. Ferrer
Abstract
A kilonova is an r-process-powered thermal transient created in the aftermath of a binary neutron star or black hole-neutron star merger. During these merger events, gravitational waves act as alert signals for telescopes to respond and search for potential optical counterparts. The localization probability regions for gravitational waves on the sky can be large. Additionally, kilonovae are rapidly-fading transients, making it crucial to develop an efficient method to detect them in image data. The standard method to detect transients is to subtract an image containing the plausible transient from a reference image of the same region taken at a different epoch. I develop a new method to detect these transients based on a particular machine learning algorithm called an artificial neural network. I trained two types of neural networks, a convolutional neural network and a dense layer network, on simulated transient samples, and tested these networks on samples created from real image data. I use images obtained by the Dr. Cristina V. Torres Memorial Astronomical Observatory and archival reference images from the Sloan Digital Sky Survey. I discuss the efficiency of each model to discriminate between transient and non-transient samples. Both models obtained high accuracy. My method does not require computationally-expensive tasks like image subtraction, and can be used on images from different telescope systems. An autonomous detection method replaces the standard process of detecting transients, normally achieved by human inspection, by a machine learning method which allows robotic telescopes to achieve greater accuracy in detecting transients, and allows for the rapid and autonomous follow-up of interesting targets of opportunity. The method will be further tested on telescopes participating in the Transient Robotic Observatory of the South Collaboration.
Recommended Citation
Wardęga, K. (2020). Using Artificial Neural Networks to Detect Astronomical Transients [Master's thesis, The University of Texas Rio Grande Valley]. ScholarWorks @ UTRGV. https://scholarworks.utrgv.edu/etd/793
Comments
Copyright 2020 Katarzyna Wardęga. All Rights Reserved.
https://www.proquest.com/docview/2452444639