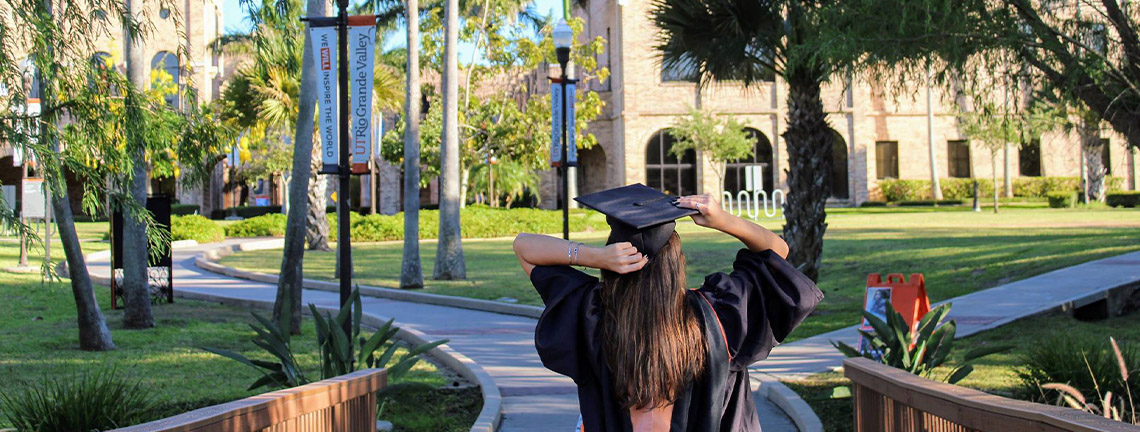
Theses and Dissertations
Date of Award
5-2021
Document Type
Thesis
Degree Name
Master of Science (MS)
Department
Electrical Engineering
First Advisor
Dr. Weidong Kuang
Second Advisor
Dr. Heinrich Foltz
Third Advisor
Dr. Jingru Zhang
Abstract
Detecting pedestrian flow in different directions at at traffic-intersection has always been a challenging task. Challenges include different weather conditions, different crowd densities, occlusions, lack of available data, and so on. The emergence of deep learning and computer vision algorithms has shown promises to deal with these problems. Most of the recent works only focus on either detecting combined pedestrian flow or counting the total number of pedestrians. In this work, we have tried to detect not only combined pedestrian flow but also pedestrian flow indifferent directions. Our contributions are, 1) we are introducing a synthetic pedestrian dataset that we have created using a videogame and a real-world dataset we have collected from the street. Our dataset has small, medium and high-density pedestrians crossing a crossroad, captured from different camera height, 2) We have proposed a Pedestrian Flow Inference Model (PFIM) that is trained on the synthetic dataset first and then is tested extensively on our real-world dataset. While testing on real-world dataset, we have embraced domain adaptation to reduce the domain gap between synthetic data and real-world data. Our proposed Pedestrian Flow Inference Model (PFIM) can detect pedestrian density and flow regardless of the height of the camera in three different ways - from left to right direction, from right to left direction, and total. Combining all, It has successfully tackled the challenges mentioned above and achieved state-of the-art performances.
Recommended Citation
Baul, Abhijit, "Learning to Detect Pedestrian Flow in Traffic Intersections from Synthetic Data" (2021). Theses and Dissertations. 829.
https://scholarworks.utrgv.edu/etd/829
Comments
Copyright 2021 Abhijit Baul. All Rights Reserved.
https://go.openathens.net/redirector/utrgv.edu?url=https://www.proquest.com/dissertations-theses/learning-detect-pedestrian-flow-traffic/docview/2564528581/se-2?accountid=7119