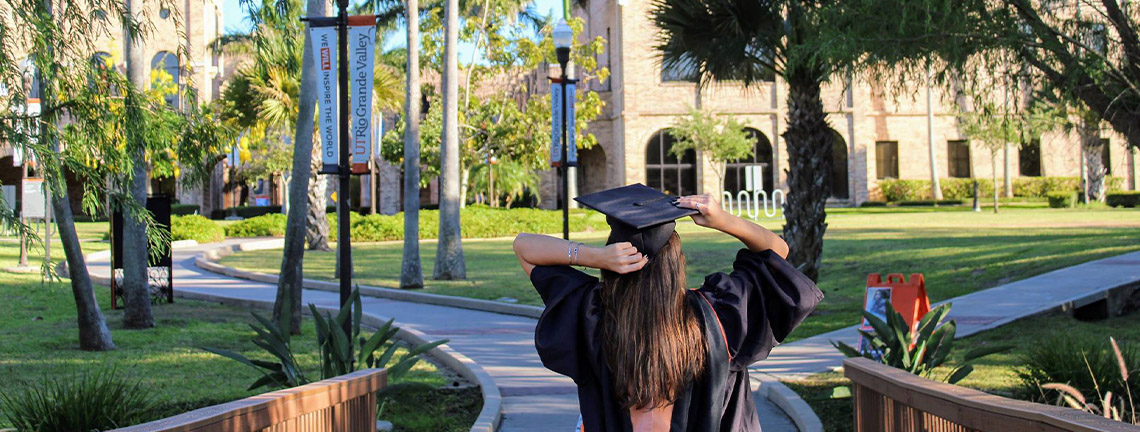
Theses and Dissertations
Date of Award
5-2022
Document Type
Thesis
Degree Name
Master of Science (MS)
Department
Civil Engineering
First Advisor
Dr. Jungseok Ho
Second Advisor
Dr. Dongchul Kim
Third Advisor
Dr. Andrew Ernest
Abstract
Texas coastal communities are at constant risk of hurricane impacts every storm season. It is especially important to model and predict storm surge variations during hurricane and storm events. Traditionally, hurricane storm surge predictions have been the result of numerical hydrodynamics based simulations. This type of simulations often requires high amounts of computational resources and complex ocean modelling efforts. Recently, machine learning techniques are being explored and are gaining popularity in hydrologic and ocean engineering modelling fields based on their performance to model nonlinear relationships and low computational requirements for prediction. Advances in machine learning and artificial intelligence (A.I.) demand the application of these methods for the modelling of complex problems such as storm surge. This study gathers historical water level data from coastal buoy stations, uses gridded forecasted weather datasets, and builds a database of ADCIRC hydrodynamic simulations to create a machine learning based surrogate model to provide timely, non-computationally intensive and accurate storm surge predictions for the Lower Laguna Madre in Texas.
Recommended Citation
Davila Hernandez, C. E. (2022). Machine Learning Based Surrogate Model for Hurricane Storm Surge Forecasting in the Laguna Madre [Master's thesis, The University of Texas Rio Grande Valley]. ScholarWorks @ UTRGV. https://scholarworks.utrgv.edu/etd/850
Comments
Copyright 2022 Cesar E. Davila Hernandez. All Rights Reserved.
https://go.openathens.net/redirector/utrgv.edu?url=https://www.proquest.com/dissertations-theses/machine-learning-based-surrogate-model-hurricane/docview/2699720602/se-2?accountid=7119