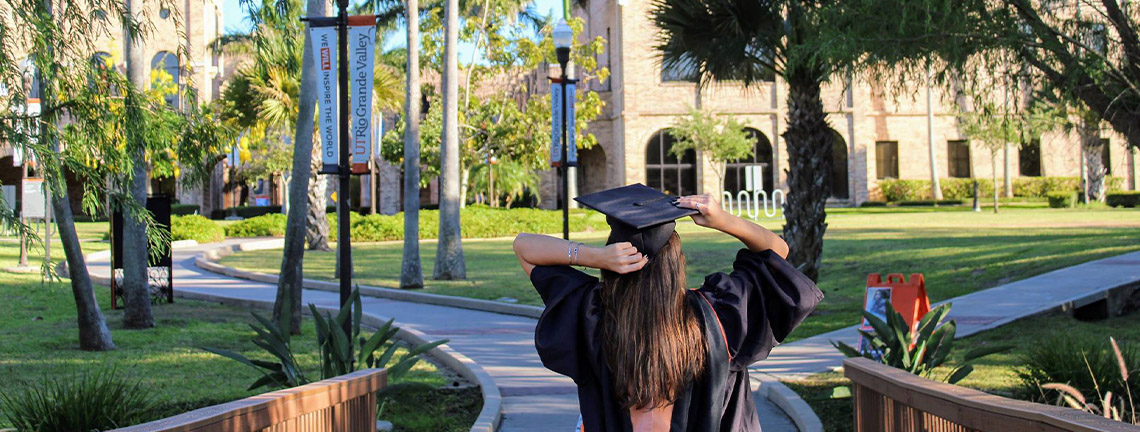
Theses and Dissertations
Date of Award
5-2022
Document Type
Thesis
Degree Name
Master of Science (MS)
Department
Mechanical Engineering
First Advisor
Dr. Isaac Choutapalli
Second Advisor
Dr. Robert Freeman
Third Advisor
Dr. Horacio Vasquez
Abstract
The coefficient of pressure distribution for various 2D airfoil geometries were found using source – vortex panel methods. The data obtained in these simulations was used in multiple machine learning models which would predict the airfoil geometry from a given coefficient of pressure distribution. The neural networks employed were fully connected feedforward networks with Levenberg – Marquardt backpropagation and one model employed Bayesian Regularization. A novel tool for optimizing airfoil shape for a given coefficient of pressure distribution was created which performed well during testing. These models serve as the first step in minimizing the conflict between aerodynamic and stealth design for both low-speed and high-speed aircraft.
Recommended Citation
Martinez, Noe Jr., "Machine Learning Based Aerodynamic Shape Optimization" (2022). Theses and Dissertations. 914.
https://scholarworks.utrgv.edu/etd/914
Comments
Copyright 2022 Noe Martinez, Jr. All Rights Reserved.
https://go.openathens.net/redirector/utrgv.edu?url=https://www.proquest.com/dissertations-theses/machine-learning-based-aerodynamic-shape/docview/2699728240/se-2?accountid=7119