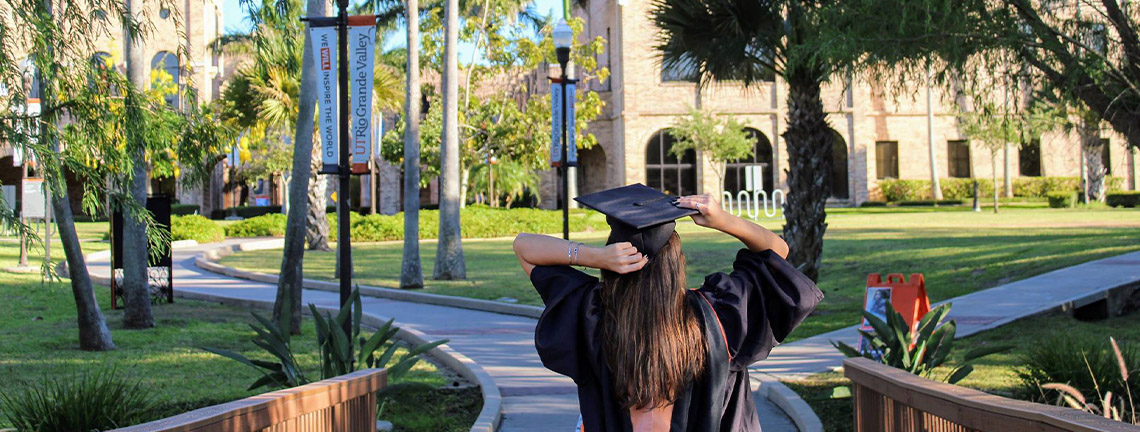
Theses and Dissertations
Date of Award
5-2021
Document Type
Thesis
Degree Name
Master of Science (MS)
Department
Computer Science
First Advisor
Dr. Hansheng Lei
Second Advisor
Dr. Fitratullah Khan
Third Advisor
Dr. Mahmoud K. Quweider
Abstract
Despite the breakthroughs in machine learning, most classifiers are not robust against adversarial attacks. They can be easily fooled by adversarial examples. These examples can be created in a variety of ways. In this thesis, the ideas of detecting edges or critical pixels in an image are investigated that could be used for fooling classifiers. Identifying those critical pixels in an image can lead the way to fix the vulnerabilities and thus making it robust against cyber-attacks. For testing, a Support Vector Machine (SVM) is used to see the success of the adversarial examples generated.
Recommended Citation
Rodriguez, Yessica, "Semantic Adversarial Attack on Support Vector Machine" (2021). Theses and Dissertations. 953.
https://scholarworks.utrgv.edu/etd/953
Comments
Copyright 2021 Yessica Rodriguez. All Rights Reserved.
https://go.openathens.net/redirector/utrgv.edu?url=https://www.proquest.com/dissertations-theses/semantic-adversarial-attack-on-support-vector/docview/2564508851/se-2?accountid=7119