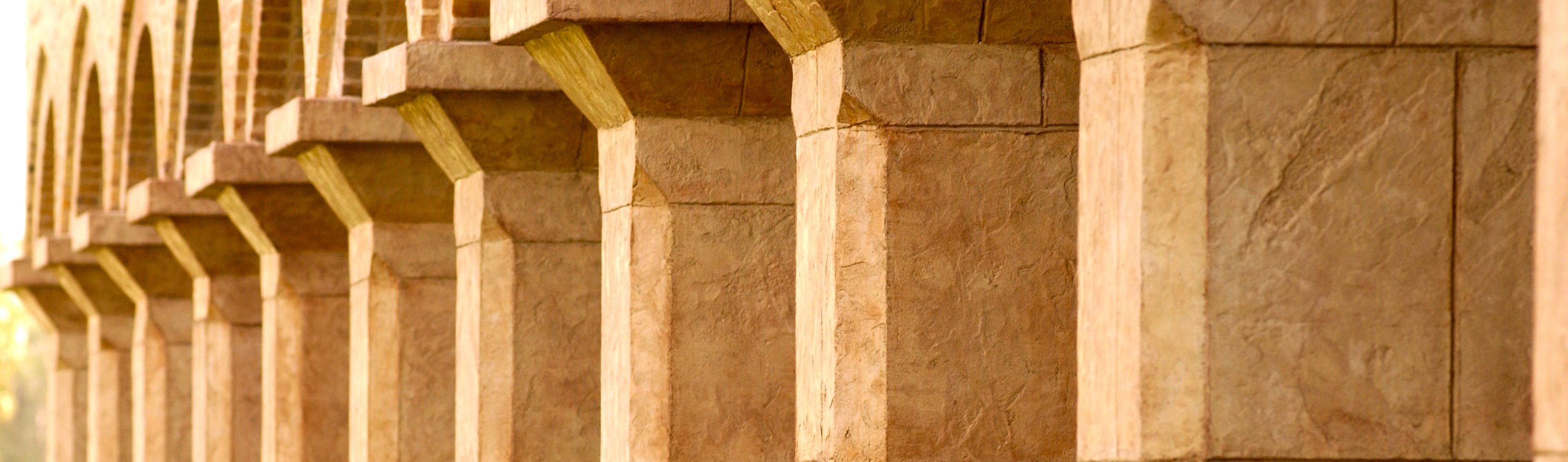
Manufacturing & Industrial Engineering Faculty Publications and Presentations
Document Type
Article
Publication Date
10-2024
Abstract
Carbon fiber-reinforced plastics (CFRP) are widely used in various industries due to their high strength to weight ratio, corrosion resistance, durability, and excellent thermo-mechanical properties. The machining of CFRP composites has always been a challenge for the manufacturers. In this study, CNC turning operation with coated carbide tool is used to machine a specific CFRP and the relationship between the cutting parameters (Speed, Feed, Depth of Cut) and response parameters (Vibration, Surface Finish, Cutting Force and Tool Wear) are investigated. An adaptive-network-based fuzzy inference system (ANFIS) model with two multi-input–single-output (MISO) system has been developed to predict the tool wear and surface finish. Speed, feed, depth of cut, vibration and cutting force have been used as input parameters and tool wear and surface finish have been used as output parameters. Three sets of cutting parameter have been used to gather the data points for continuous turning of CFRP composite. The model merged fuzzy inference modeling with artificial neural network learning abilities, and a set of rules is constructed directly from experimental data. This model is capable of predicting the cutting tool wear and surface finish during turning of CFRP composite. The predicted tool wear and surface finish data are compared to the experimental results. The predicted data agreed well with the actual experimental data with 98.96 % accuracy for tool wear and 99.61 % accuracy for surface finish.
Recommended Citation
Srivastava, Anil K., and Md Mofakkirul Islam. "Prediction of tool wear and surface finish using ANFIS modelling during turning of Carbon Fiber Reinforced Plastic (CFRP) composites." Manufacturing Letters 41 (2024): 658-669. https://doi.org/10.1016/j.mfglet.2024.09.084
Creative Commons License
This work is licensed under a Creative Commons Attribution-NonCommercial-No Derivative Works 4.0 International License.
Publication Title
Manufacturing Letters
DOI
https://doi.org/10.1016/j.mfglet.2024.09.084
Comments
© 2024 The Authors. This is an open access article under the CC BY-NC-ND license (https://creativecommons.org/licenses/by-nc-nd/4.0)