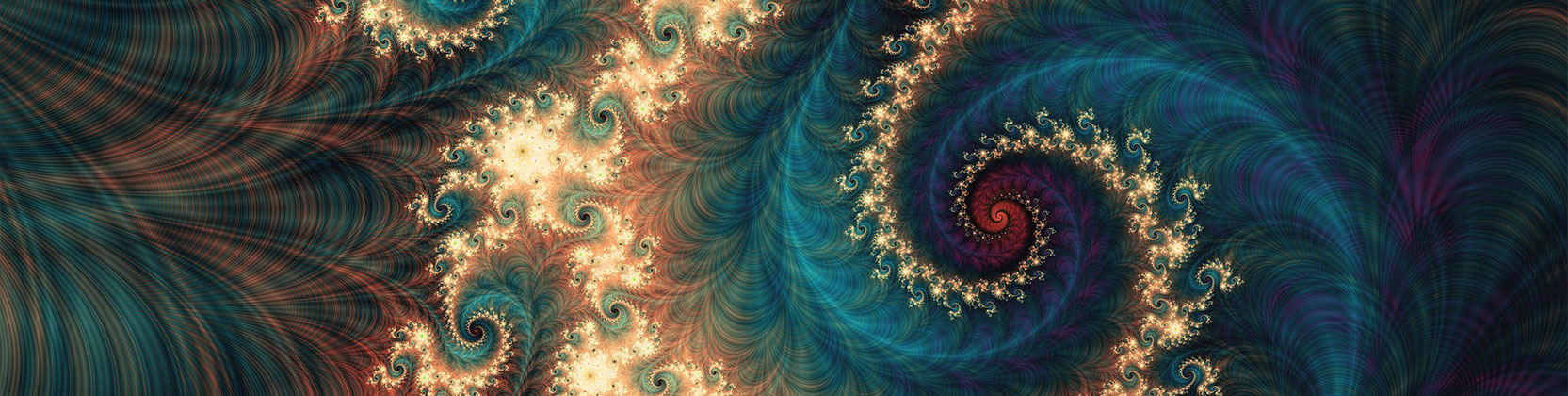
School of Mathematical and Statistical Sciences Faculty Publications and Presentations
Document Type
Article
Publication Date
2-8-2023
Abstract
The parameters of the log-logistic distribution are generally estimated based on classical methods such as maximum likelihood estimation, whereas these methods usually result in severe biased estimates when the data contain outliers. In this paper, we consider several alternative estimators, which not only have closed-form expressions, but also are quite robust to a certain level of data contamination. We investigate the robustness property of each estimator in terms of the breakdown point. The finite sample performance and effectiveness of these estimators are evaluated through Monte Carlo simulations and a real-data application. Numerical results demonstrate that the proposed estimators perform favorably in a manner that they are comparable with the maximum likelihood estimator for the data without contamination and that they provide superior performance in the presence of data contamination.
Recommended Citation
Ma, Zhuanzhuan, Min Wang, and Chanseok Park. "Robust explicit estimation of the log-logistic distribution with applications." Journal of Statistical Theory and Practice 17, no. 2 (2023): 21. https://doi.org/10.1007/s42519-023-00322-x
Publication Title
Journal of Statistical Theory and Practice
DOI
10.1007/s42519-023-00322-x
Comments
Original published version available at https://doi.org/10.1007/s42519-023-00322-x