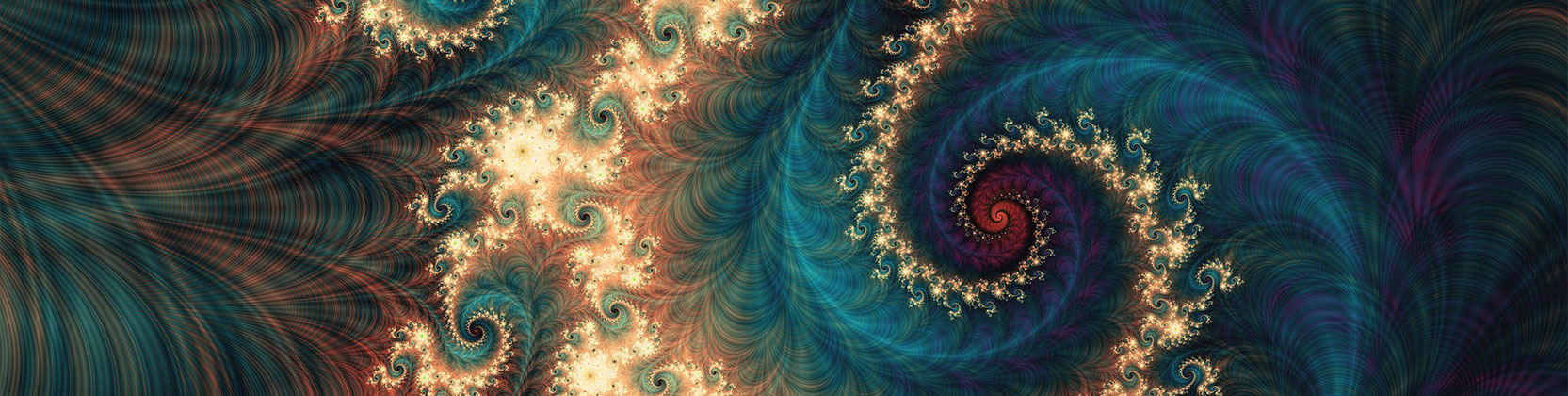
School of Mathematical and Statistical Sciences Faculty Publications and Presentations
Bayesian variable selection and estimation in binary quantile regression using global-local shrinkage priors
Document Type
Article
Publication Date
12-11-2023
Abstract
In this paper, we construct a Bayesian hierarchical model with global-local shrinkage priors for the regression coefficients, which includes the horseshoe prior and normal-gamma prior. This model is used for high-dimensional quantile regression models with dichotomous response data. We have developed an efficient sampling algorithm to generate posterior samplings for making posterior inference. We use a location-scale mixture representation of the asymmetric Laplace distribution. We assess the performance of the proposed methods through Monte Carlo simulations and two real-data applications in terms of parameter estimation and variable selection. Numerical results demonstrate that the proposed methods perform comparably with existing Bayesian methods under a variety of scenarios.
Recommended Citation
Zhuanzhuan Ma, Zifei Han & Min Wang (2023) Bayesian variable selection and estimation in binary quantile regression using global-local shrinkage priors, Communications in Statistics - Simulation and Computation, https://doi.org/10.1080/03610918.2023.2293638
Publication Title
Communications in Statistics - Simulation and Computation
DOI
10.1080/03610918.2023.2293638
Comments
© 2023 Taylor & Francis Group, LLC.
https://www.tandfonline.com/share/YQKXUCF9IGNXRXSXEHAT?target=10.1080/03610918.2023.2293638