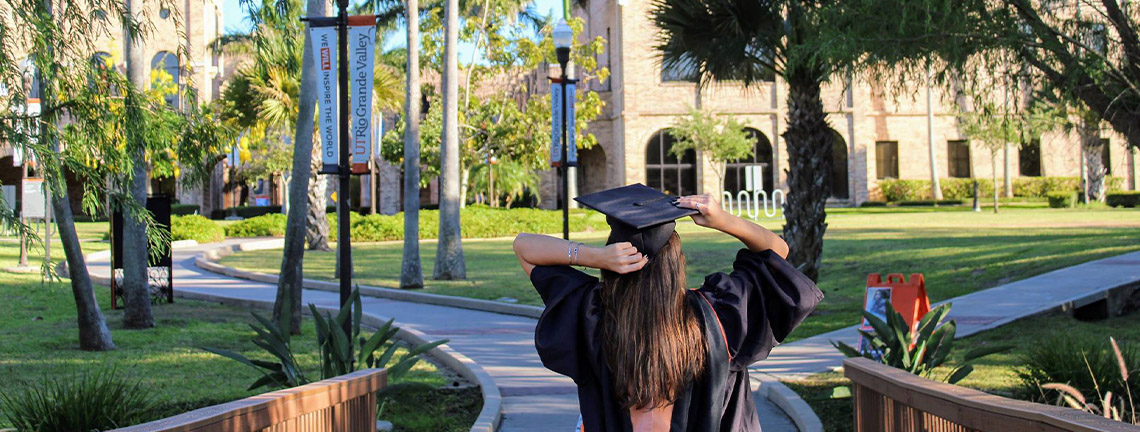
Theses and Dissertations
Date of Award
8-1-2024
Document Type
Thesis
Degree Name
Master of Science (MS)
Department
Mathematics
First Advisor
Tamer Oraby
Second Advisor
Michael Machiorlatti
Third Advisor
Hansapani Rodrigo
Abstract
In the repeated measures longitudinal datasets where missing data is a relatively common issue, we explored different imputation methods, including machine learning (ML) methods in order to examine potential efficiencies for using traditional versus newer computational methods. In order to accomplish said comparison, we used a Monte Carlo simulation experiment of a population of size N=70000 to mimic a clinical trial, with different scenarios of missing at random (MAR) data in the response variable. To compare the behavior of each method resulting from the difference between population and sample dataset, a real dataset was used from the Boston College on "National Longitudinal Survey" to simulate MAR. Moreover, we used both datasets to examine the effects of different sample sizes when using Bayesian neural networks and k-Nearest Neighbors (k-NN) for imputations and compared that to the more traditional methods of last observed carried forward, multiple imputation, and linear regression. Additionally, the cost of computing power is evaluated at different sample sizes for each scenario on both datasets.
Recommended Citation
Alanis, J. O. (2024). Missing Data Imputation With Longitudinal Data [Master's thesis, The University of Texas Rio Grande Valley]. ScholarWorks @ UTRGV. https://scholarworks.utrgv.edu/etd/1589
Comments
Copyright 2024 Joseph O. Alanis. https://proquest.com/docview/3116494195