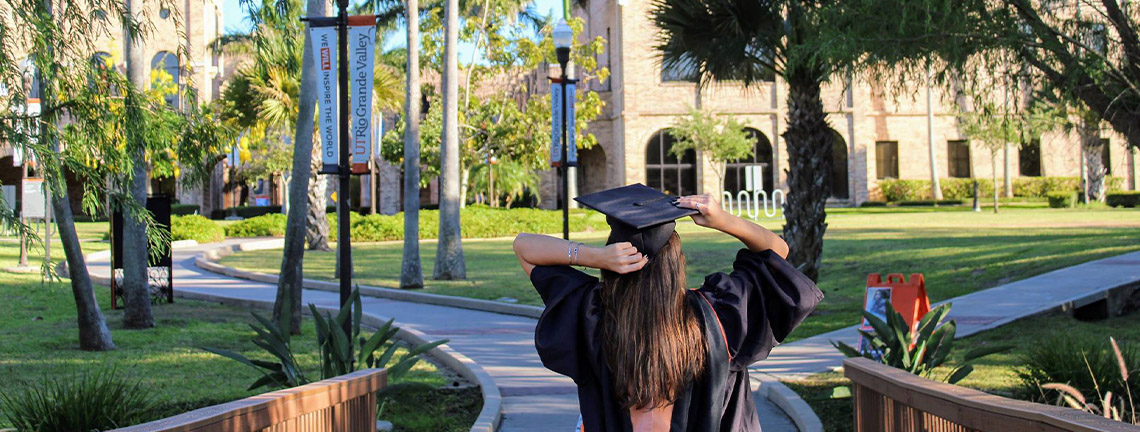
Theses and Dissertations
Date of Award
5-2024
Document Type
Thesis
Degree Name
Master of Science (MS)
Department
Applied Statistics and Data Science
First Advisor
George P. Yanev
Second Advisor
Tamer F. Oraby
Third Advisor
Santanu Chakraborty
Abstract
The Generalized Poisson distribution is useful in modeling epidemiological processes as a branching stochastic processes problem. Our goal is to construct accurate and reliable estimators for the reproduction number (R0) (i.e., the number of secondary infections), particularly in the context of disease outbreaks modeled by a Galton-Watson process. Towards this goal, we construct the classical Bayes estimator, the Maximum Likelihood estimator, and the Empirical Bayes (EB) estimator under the Square Error Loss function in Chapter II. We prove that the Empirical Bayes estimator is asymptotically optimal and estimate the rate of convergence. We then proceed to monotonize the Empirical Bayes estimator in Chapter III using the Van Houwelingen method [22] and the Isotonic Regression method [3], then introduced the concept of the risk and regret risk associated with our estimators. For the numerical study in Chapter IV we assume a Poisson distribution for the reproduction number and that the initial number of infected individuals follows a Poisson distribution. Simulation results indicate that the empirical estimator suffers from "jumpiness", hence the need for monotonization. We then compare the regret risks of each of the estimators and find out that the monotonized estimate outperforms the others.
Recommended Citation
Johnson, Alberta Araba, "Bayesian Estimation of Reproduction Numbers from Distributions of Outbreaks Sizes: Branching Process Approach" (2024). Theses and Dissertations. 1517.
https://scholarworks.utrgv.edu/etd/1517
Comments
Copyright 2024 Alberta Araba Johnson.
https://go.openathens.net/redirector/utrgv.edu?url=https://www.proquest.com/pqdtglobal1/dissertations-theses/bayesian-estimation-reproduction-numbers/docview/3085283692/sem-2?accountid=7119